The field of artificial intelligence (AI) and machine learning (ML) is continually evolving, driven by advances in technology, algorithms, and data availability. As we progressed into 2020, many predictions emerged about the key research tracks and innovations that would shape the coming decade. This overview reflects on the anticipated trends in AI and ML, drawing insights from the research landscape at the start of the 2020s.
1. Graph Machine Learning.
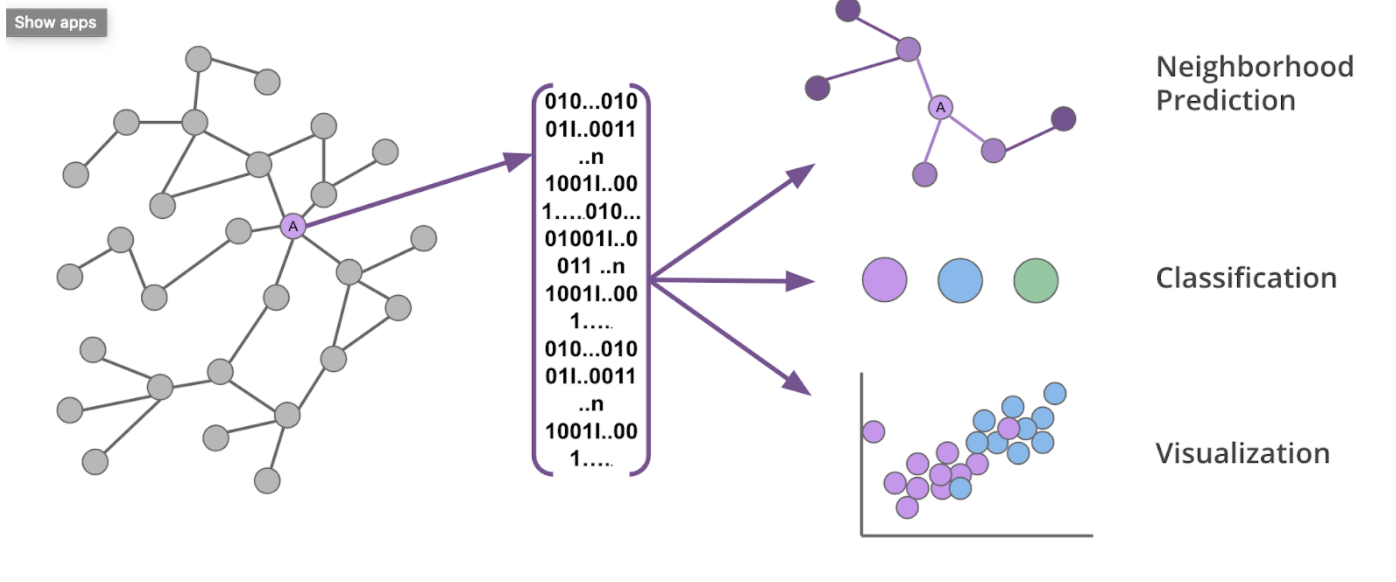
- Overview: Graph machine learning involves the application of machine learning techniques to graph-structured data. Given the inherent relationships in data, graphs provide a powerful way to represent and analyze information.
- Future Outlook: Researchers were expected to develop more sophisticated algorithms for graph-based learning, enabling improvements in social network analysis, recommendation systems, and bioinformatics. The continued integration of graph theory into AI could lead to breakthroughs in understanding complex systems and interactions.
2. Advancements in Convolutional Neural Networks (CNNs).
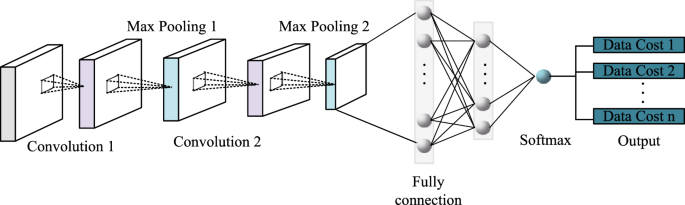
- Overview: CNNs have dominated the computer vision landscape since their inception, proving effective in image classification, object detection, and segmentation tasks.
- Future Outlook: Expectations included enhancements in CNN architectures, leading to greater accuracy and efficiency. Researchers were likely to focus on optimizing models to run on edge devices, enabling real-time processing in applications such as autonomous vehicles and smart cameras. This trend towards lightweight models could democratize access to powerful AI capabilities
3. Semi-supervised Learning.
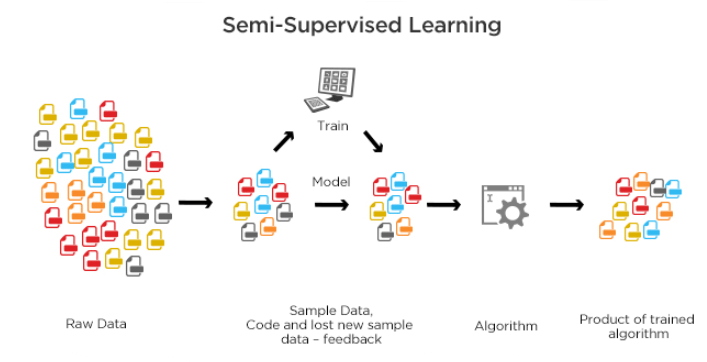
- Overview: Semi-supervised learning bridges the gap between supervised and unsupervised learning, utilizing both labeled and unlabeled data to improve model performance. This approach is particularly valuable given the high costs and time associated with data labeling.
- Future Outlook: The trend towards semi-supervised learning was anticipated to gain momentum, particularly in applications where labeled data is scarce. Researchers were expected to refine techniques that effectively leverage vast amounts of unlabeled data, driving advancements in natural language processing (NLP) and image recognition tasks. This could lead to more robust models capable of generalizing better in diverse environments.
4. Generative Models.
- Overview: Generative models, including Generative Adversarial Networks (GANs) and Variational Autoencoders (VAEs), allow the generation of new data points from learned distributions, enabling creativity in content generation and data augmentation.
- Future Outlook: The field was poised for significant growth, with expectations for more stable and scalable generative models. Researchers were likely to explore applications in art, music, and synthetic data generation, as well as in fields like healthcare, where generative models could assist in drug discovery and personalized medicine.
5. Handling Anomalies and Adversarial Attacks.
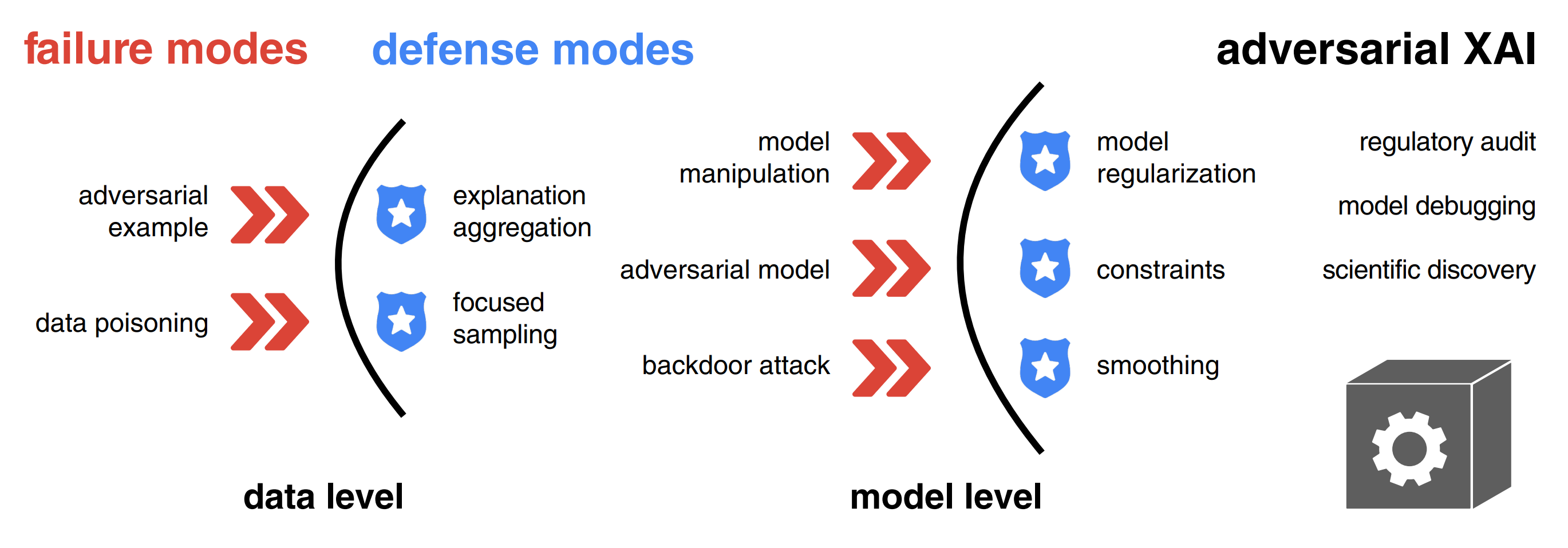
- Overview: As AI systems become more pervasive, the challenges of detecting anomalies and defending against adversarial attacks are increasingly critical. Anomalies can signal fraud, security breaches, or equipment malfunctions, while adversarial attacks exploit vulnerabilities in models to deceive them.
- Future Outlook: Research efforts were expected to intensify around robust model training and validation techniques that enhance the resilience of AI systems against such threats. Innovations in this area could lead to safer AI applications, particularly in sensitive domains like finance, healthcare, and autonomous systems.
6. Scalability and Efficiency.
- Overview: Scalability refers to the ability of AI systems to handle growing amounts of data and complexity, while efficiency relates to the computational resources required for training and inference.
- Future Outlook: The demand for AI solutions that can operate at larger scales while minimizing resource consumption was anticipated to rise. Researchers were expected to focus on distributed learning, model compression techniques, and federated learning approaches that allow for decentralized training on multiple devices while preserving privacy.
7. AI for Social Good.
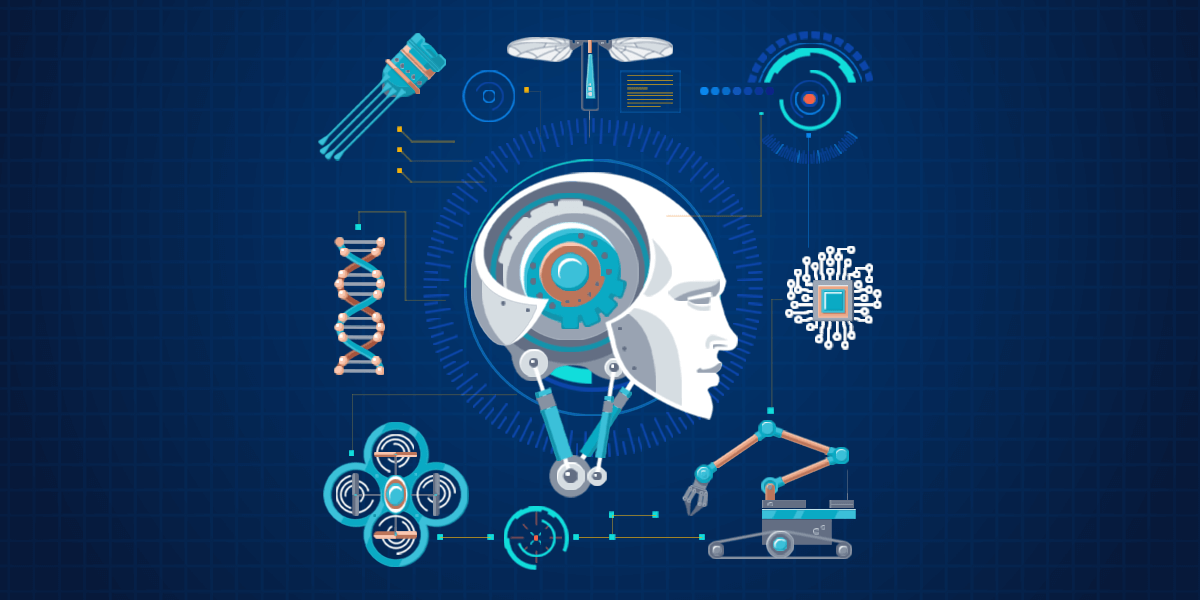
- Overview: The potential of AI to address global challenges, from climate change to public health, was becoming increasingly recognized.
- Future Outlook: There was a growing expectation for researchers and organizations to develop AI applications aimed at promoting sustainability, improving healthcare access, and enhancing educational opportunities. Collaborations between academia, industry, and non-profits were expected to create impactful solutions that leverage AI for positive social change.
8. Explainable AI (XAI).
- Overview: As AI systems become more complex and influential in decision-making, the need for transparency and interpretability has become crucial. Explainable AI seeks to make the operations of AI systems understandable to humans.
- Future Outlook: Researchers were likely to prioritize the development of methods that not only enhance model performance but also provide insights into how decisions are made. This focus on explainability will be essential for building trust in AI applications, especially in regulated industries like finance and healthcare.
9. Transfer Learning and Domain Adaptation.
- Overview: Transfer learning involves taking a pre-trained model developed for one task and fine-tuning it for a different but related task. Domain adaptation focuses on adjusting models to work effectively across different datasets or environments.
- Future Outlook: The application of transfer learning was expected to grow, particularly in situations where labeled data is limited. Researchers were likely to explore more effective techniques for adapting models to new domains, enabling faster deployment of AI solutions in various fields, from robotics to customer service.
10. Human-AI Collaboration.
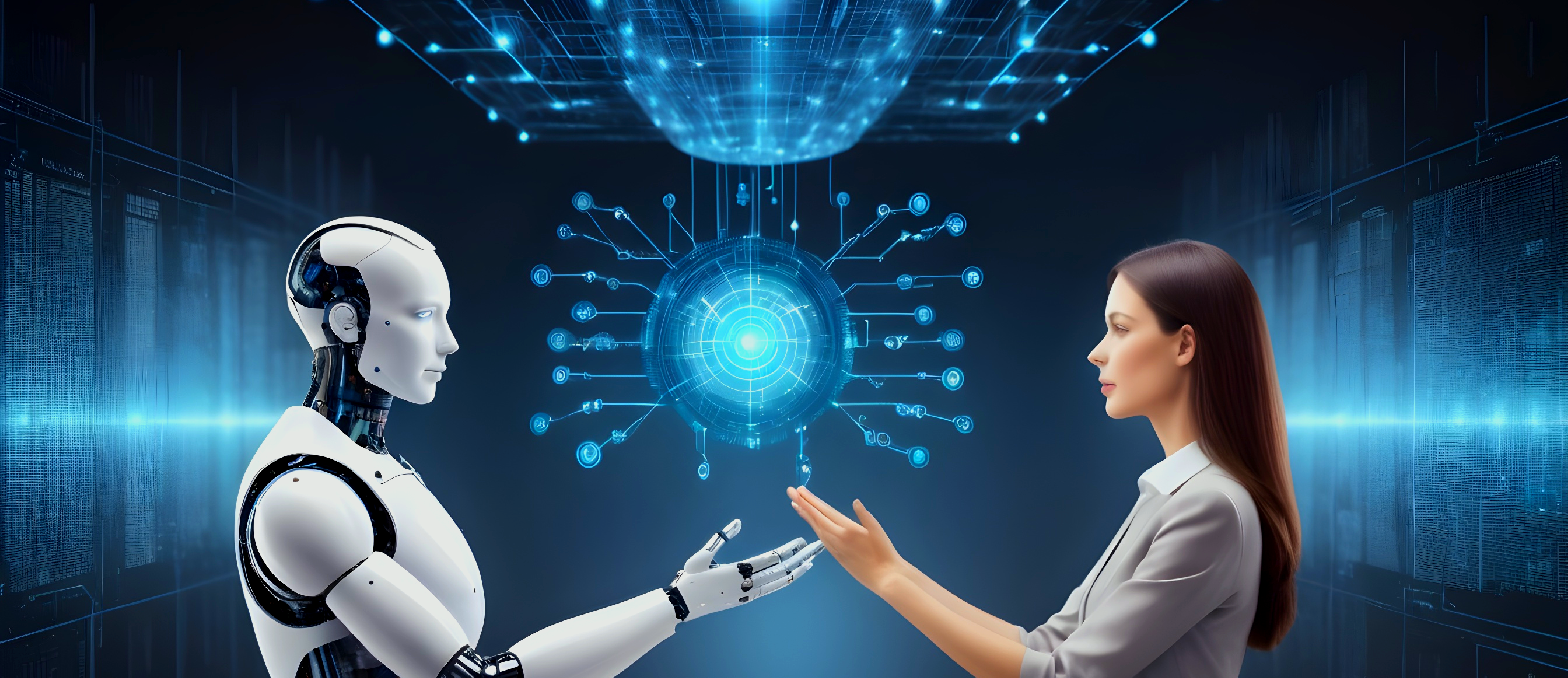
- Overview: The relationship between humans and AI systems is evolving from one of dependency to collaboration, where AI assists humans in decision-making and creative processes.
- Future Outlook: Future research was expected to emphasize developing AI systems that complement human skills and capabilities, fostering synergy between human intelligence and artificial intelligence. This could lead to new paradigms in various sectors, including healthcare, where AI might assist doctors in diagnosis and treatment planning, or in creative fields, where AI tools could help artists and writers explore new avenues of expression.
Conclusion
The landscape of artificial intelligence and machine learning at the turn of the decade was characterized by rapid advancements and a growing understanding of how to leverage these technologies effectively. Predictions made about the future of AI and ML reflect the collective aspirations of researchers and practitioners to address complex challenges and drive innovation across multiple sectors.
As we move further into the 2020s, the trends highlighted above offer a glimpse into the potential directions that research and application may take. With the continuing evolution of AI and ML, the focus on ethical considerations, scalability, explainability, and real-world impact will be crucial in shaping a future where AI technologies benefit society as a whole. By harnessing the power of data, fostering collaboration, and embracing experimentation, the AI community stands poised to unlock new possibilities and create a positive legacy for future generations.
Comments (0)